Features:
GDELT and the Problem of Decontextualized Data
How FiveThirtyEight Got the Nigerian Kidnappings Analysis Wrong
On the evening of April 14, more than 100 schoolgirls were kidnapped in Chibok, a small town in northeast Nigeria. Days later, the reported number of abducted girls increased to 200, and almost immediately returned to 130, an official count. On April 21, the parents of Chibok increased the number yet again, to 234. One month after the initial event, international media reports have settled on 276 abductees. More than 70 girls escaped their captors; over 200 girls remain missing.
These are not simply numbers. Each represents a victim—and, one hopes, a survivor—of the mass violence of Boko Haram, the insurgency that, alongside their military adversaries, has razed much of Nigeria’s northeasternmost Borno state since 2009. Both Boko Haram and the evolution of their violence are difficult to understand, even for veteran students of Nigeria’s politics. Like many insurgencies, Boko Haram is opaque; until recently, neither its decision-making nor its leadership, nor the way the organization retains its recruits, were clear. Consequently, northern Nigeria’s violence is data-poor. The violence is known by those who experience it, but it is scarcely observed by outsiders.
Since the abduction of the Chibok girls, as well as the global campaign—“Bring Back Our Girls”—to ensure their rescue, international journalists have sought to make sense of Boko Haram’s violence. Most commonly, journalists convey the stories of the insurgents’ victims: mothers and fathers and daughters who, in Chibok’s wake, struggle to re-patch an ever-broken community. Others—analysts of violent conflict, mostly—use aggregated, often quantitative data to describe the conflict’s scope. To paraphrase Mahmood Mamdani, these analysts strive to make the violence thinkable, if never fully comprehensible.
FiveThirtyEight’s Stories
Chief among this latter group is data journalism site FiveThirtyEight. Since the Chibok crisis began, Mona Chalabi, a lead writer at the site’s DataLab, has published two posts describing Nigerian kidnapping trends between the early 1980s and the present. Chalabi’s posts use the Global Data on Events, Language, and Tone (GDELT)*, an automated catalog of global political events as reported in the media, to identify discrete kidnappings throughout Nigeria’s contemporary history. At first glance, the data–and the visualizations that follow–are astonishing: allegedly, “[t]here have been 2,285 kidnappings in Nigeria in the first four months of 2014.” On the day the Chibok crisis began, April 14, “the database records 151 kidnappings.”
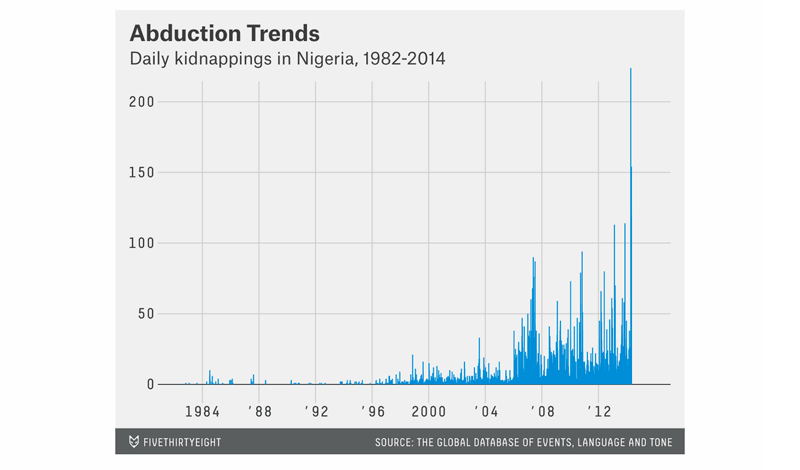
from FiveThirtyEight’s Kidnapping of Girls in Nigeria Is Part of a Worsening Problem
Something is awry in Chalabi’s analysis. Despite its global media spotlight, the Chibok abduction doesn’t tell us very much about Boko Haram’s operations. Kidnapping is one among several tactics, including armed robbery and criminal extortion, that Boko Haram uses to raise funds, and the group is more likely to use lethal violence than kidnapping to intimidate civilians. From a relative perspective, other Nigerian insurgencies–such as the Movement for the Emancipation of the Niger Delta (MEND), which was active throughout the 2000s–have used kidnapping much more frequently than has Boko Haram. Chalabi’s time-series visualization disagrees: the average daily kidnapping count between 2000 and 2009 is significantly lower than during the first five months of 2014. To describe the depicted trend as unlikely would be a grave understatement.
Chalabi’s analytic misstep stems from her misuse of the GDELT dataset. Here, Erin Simpson, a Washington, DC-based quantitative social scientist who has worked closely with the GDELT community, puts it best:
All trend analysis using #GDELT has to take into account the exponential increase in news stories which generate the data. @FiveThirtyEight
— EM Simpson (@charlie_simpson) May 13, 2014
#GDELT isn't designed for tracking discrete events like "kidnappings" or "suicide bombings" bc it's based on news reports. @FiveThirtyEight
— EM Simpson (@charlie_simpson) May 13, 2014
So if #GDELT says there were 649 kidnappings in Nigeria in 4 months, WHAT IT'S REALLY SAYING is there were 649 news stories abt kidnappings.
— EM Simpson (@charlie_simpson) May 13, 2014
(Simpson’s full and illuminating series of tweets is collected at Storify: If A Data Point Has No Context, Does It Have Any Meaning?)
Where It Went Wrong
If one looks at Chalabi’s visualizations–the time series, and then the heat map–it is easy to see where FiveThirtyEight’s analysis falls short. As Simpson notes, GDELT captures our–AP’s, or AFP’s, or Xinhua’s–observation of a political event, rather than the occurrence of the event itself. While the Chibok crisis only occurred once, GDELT counts 151 simultaneous reports; whatever its scale, the GDELT count significantly distorts the event’s meaning. In an update to the first post, Chalabi attempts a quick fix: she normalizes the kidnapping data against the full daily GDELT event count, to provide a sense of scale. By comparing a specific event to a global dataset of reports, we learn a great deal about biases in international media reporting. Unfortunately, we learn very little about specific trends in Nigerian kidnapping.
Chalabi’s second post, in which she maps Nigerian kidnapping events, also displays the raw GDELT data’s imperfections. According to the post’s heat map, among the most frequently recurring kidnapping locations is Nigeria’s central Kaduna state–a source of violence, undoubtedly, but rarely on the scale of northern Nigeria’s Borno or Kano states. As Simpson again implies, the Kaduna spot is a centroid, the primary default geolocation in Nigeria when no other location data can be found; another red herring. GDELT only captures the information that international media reports publish. In a data-poor environment like Nigeria’s, the natural-language processing that generates the reported location is no more certain than a coin toss. Chalabi does not clarify this data bias in either post.
The Wider Angle
Neither GDELT nor FiveThirtyEight are unique in the limits of their Nigeria data, nor of the analysis they provide. Recently, the Armed Conflict Location and Event Data Project (ACLED), a database of political violence events, compiled a brief comparison of Boko Haram and the Lord’s Resistance Army, and encountered similar problems with data and context. ACLED is a common resource for conflict researchers; for this effort, the project’s analysts piles violent event counts alongside civilian fatalities to demonstrate new “non-traditional” types of political violence. Placed out of context and connected only by implicit correlation, neither event counts nor civilian fatalities describe much about the other. Like FiveThirtyEight’s GDELT analysis, the ACLED effort lacks a transparent method for assessing Nigeria’s violence.
Large event datasets like GDELT do not create information; they only aggregate reporting from existing sources. As Erin Simpson observes, GDELT is only as perfect as its sources allow. Paradoxically, this imperfection makes the GDELT’s knowledge least effective in the areas, like Nigeria, where it is needed most. At a microscopic level, aggregated data reveals very little that a reported dispatch does not. But as the scope of analysis grows, perspectives like FiveThirtyEight’s become more valuable. A broader view, however, is not always synonymous with a more comprehensive one. For data journalists covering violent conflict, the imperfection of a dataset like GDELT places an additional burden on analytic transparency. In Nigeria, as elsewhere in conflict, the data’s uncertainty may prove a more fruitful story than its hasty conclusions.
* GDELT, as an institutional project, is not without controversy. In early January, John Beieler, Patrick Brandt, and Philip Schrodt, three of the project’s primary architects, severed their relationship with the dataset and its co-founder, Kalev Leetaru. The circumstances of the group’s dissociation remain unknown.
Corrections: An earlier version of this article expanded GDELT as the Global Data of Events, Location, and Tone. The L stands for Language, not Location. Additionally, a previously corrected footnote incorrectly stated a copyright dispute regarding one of the cited datasets. We regret the error.
Credits
-
Daniel Solomon
Daniel Solomon is a writer based in Washington, D.C. He has written for The New York Times, The Awl, The Week, and Pacific Standard. He blogs at Securing Rights, and you can follow him on Twitter @Dan_E_Solo.