Learning:
Human-Assisted Reporting Gets the Story
Tyler Dukes on combining the power of data-sorting tools with old-fashioned digging
In early 2013, WRAL News and other outlets in North Carolina began covering problems with a new computerized social services system launched to streamline the process of delivering benefits like food stamps.
Based on what we heard, it was far from streamlined. Food stamp clients said their benefits were weeks and months overdue, leaving a lot of hungry families with few options.
Officials from the state health department and their county counterparts were guarded in their responses. They mostly stuck to talking points about how the system required training and was a new way of doing business. It was never about bugs in the system, they said.
But that story didn’t seem to add up.
I made public records requests to the state’s health department, but was frustrated by delays (requests I made in August still haven’t been fulfilled). So I turned to a few counties to get emails of conversations between some of the top health officials regarding the performance of the system, dubbed NC FAST.
What I received a month later from Nash County, N.C., were two boxes filled with thousands of printed pages of emails. Double-sided.
Worse still, they were randomly organized. Because the county has no email archive software, IT workers physically went to each official’s computer, searched Outlook for keywords, printed the collection and hand-redacted them before dropping off the mish-mash to me.
So I turned to Overview.
Enter Overview
Created by a team at the Associated Press and initially supported by a grant from the Knight News Challenge, Overview is designed to help reporters sift through massive document sets by sorting them into piles based on similarity. That “similarity” is basically an attempt by Overview’s underlying algorithms to compare the frequency of words in each document to the frequency of those words in the entire document set. If two emails share a bunch of the same words that aren’t as common in the entire batch of emails, the assumption goes, they’re likely to be similar.
But the algorithms, being no more than dumb code without the aid of context or months spent on the beat, often get it wrong. That’s why there’s a built-in system allowing users to quickly tag individual documents (or entire piles) however the reporters want.
It sounded perfect, but first I had to digitize all 4,500 pages of emails.
Scanning It
I spent the morning hogging our station’s bulky multi-function printer to scan everything to PDF. I then uploaded them to DocumentCloud, another reporting power tool I lean on pretty heavily in my day-to-day work.
Among its other wonderful features, DocumentCloud takes every file you upload and runs optical character recognition software, translating printed pages into searchable, digital text. OCR isn’t perfect (and if your scans are messy it’s outright lousy), so it’s important to be wary of mistakes.
But because DocumentCloud is fully integrated with Overview, all I had to do is log in and import the entire document set with a click.
One important point: check the option that prompts Overview to split your project into individual pages. The program needs individual documents (emails in this case) to run its comparisons, and because there’s no easy way to break up a scanned batch like this into its component parts, this is a good alternative.
Then the real work began.
Getting to Analysis
Overview sorts the documents, but it’s still up to me to analyze them and make sense of what I find. And I really had no idea what I was looking for.
But when you’re fishing, any review of email starts with the basic assumption that 90 percent of what you’re reading will be totally useless information. There are duplicates and forwards, bureacratic language and meeting requests that will slow you down and never help your story.
That’s why Overview is so useful.
Here’s How It Works
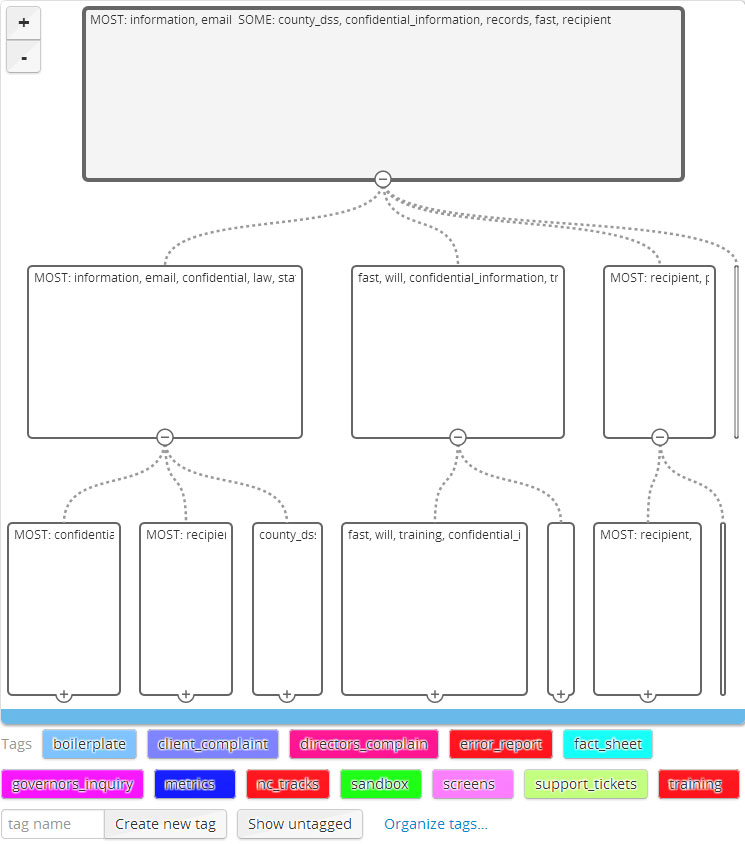
The Document Tree view on the left side of the Overview display provides a visual hierarchy that allows users to expand or collapse piles of documents sorted based on similarity.
By sorting content into computer-generated piles, you can easily dismiss a lot of the crap. Using one of my tags—“boilerplate”—I was able to rule out hundreds of pages sorted into piles with confidentiality notices and other email signatures.
So don’t be afraid to skip. You can even designate a tag like “skipped” and label a pile after reading only half of it. You’re out to get the overall tenor of the collection as efficiently as possible.
And you can always come back later. Overview has a fantastic option called “show untagged,” which filters out the piles you’ve already labeled. Looking back, I should have created a simple tag called “reviewed” to note which piles I had already seen.
When you first import a document set though, what you see is a basic tree structure with no tags. At the top, all the documents are grouped by the most common words. Clicking through the branches and sub-branches, you can expand the piles into smaller and smaller collections—each with a display of most used words—until you see something manageable.
In this case, I started four or five levels down, where the piles were narrowed to collections of a few hundred emails.
I typically start with the smallest ones, because a smaller pile theoretically means they’re the most unique documents.
Finding the Story
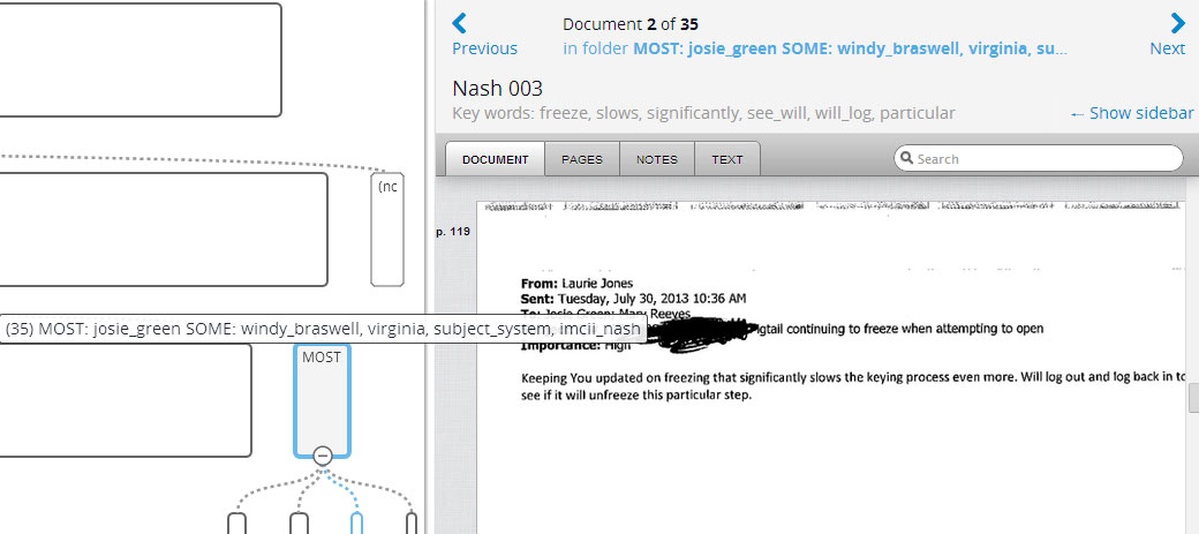
This small pile of 35 documents was a good place to start, since it means the common words here aren’t that common in the rest of the collection. It turned up a particular issue with system freezes, but I wasn’t sure yet whether this meant anything.
The first collection of 35 pages, which I could browse through via the DocumentCloud interface, wasn’t definitive. But the emails did indicate a problem workers seemed to be having with the system, which was freezing and forcing them to log out.
That seemed promising, so I kept digging.
After a few hours, I was able to detect a similar problem over and over again.
Then, in a collection of 147 documents four levels down the tree structure, I found something really interesting.
Unknown to me at the time, one of the county workers whose emails I requested was a member of a statewide organization of social services directors. And that group had a listserv.
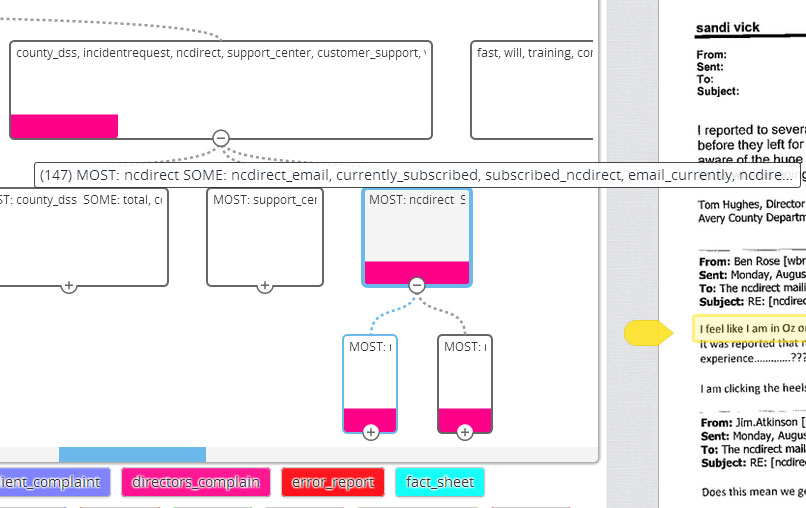
Here was the most useful pile—a trove of listserv emails largely complaining of big problems with the state’s software.
Over the course of a month and a half, directors from dozens of counties had vented to each other about the problems their staff members were seeing with the NC FAST system and the lack of support and acknowledgement they were getting from the state.
“It is time for the state to get out in front of this,” one county worker wrote to his colleagues. “Statements to date of minor problems, worker error and requests for patience now sound empty and insulting.”
Up until this point, we had never seen that level of candor from anyone at the county or state level.
Overview grouped all of these messages—spread over weeks of correspondence and multiple email accounts—in one single pile. And thanks to the integration of DocumentCloud, everything I found could be annotated so I could later show my work to editors and readers.
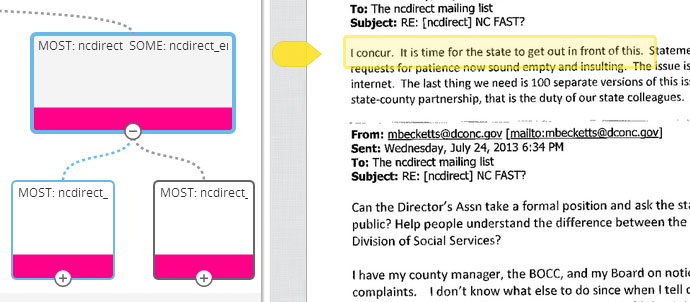
Because Overview is fully implemented with DocumentCloud, I was able to annotate these documents quickly when I found something interesting. I eventually used these annotations as clickable links in the story, allowing readers to see the larger context.
What We Found
Combined with other public statements and interviews from the state, we were able to show in our December story that the N.C. Department of Health and Human Services downplayed widespread technical problems with the new system, frustrating county workers who were all but begging the state to acknowledge the problem.
The bug ended up being a browser compatibility problem with Internet Explorer that took almost two months to fix, and even then the solution was to switch to Google Chrome.
Meanwhile, counties built up a backlog of overdue food stamp cases that totaled almost 70,000—8.5 percent of the families the state serves every month.
It’s possible I could have found those emails the old fashioned way, by sorting through piece by piece until I found something interesting.
But given that I was able to get a good handle on what I had in an afternoon—on my own—I can’t help but think this method of “human-assisted reporting” was far more effective than devoting the time and resources to do everything by hand.
Stories like these are important, and I’m glad services like Overview and DocumentCloud exist to help us tackle them.
Credits
-
Tyler Dukes
Tyler is an investigative reporter at The News & Observer in Raleigh, N.C., specializing in data and public records. He also teaches courses on data journalism at the DeWitt Wallace Center for Media & Democracy at Duke University’s Sanford School of Public Policy. In 2017, he completed a Nieman Fellowship at Harvard University. Prior to joining the N&O, Tyler worked as an investigative reporter at WRAL and was the managing editor for the Duke University Reporters’ Lab, a research project aimed at reducing the costs of investigative reporting.